Furthering Experimentation Practice - Part II: Process & Pathways
A rigorous experimentation approach (experiment-to-learn) will most likely outperform planning-based approaches (analyse-to-predict) where the challenge is dynamic and ever changing (i.e. any complex social and environmental challenge). This post explores how to do experimentation for change.
In Furthering Experimentation Practice - Part I, we talked about framing experimentation in the context of Directionality, and in the context of the dynamics of how change happens.
As I've been writing this series, I realise that I see experimentation as a generic practice which can be adopted into a range of different change processes, as an adaptive approach to both implementation and governance - optimising for learning and adaptation in response to complex challenges, rather than prediction and control.
A rigorous experimentation approach (experiment-to-learn) will most likely outperform planning-based approaches (analyse-to-predict) where the challenge is dynamic and ever changing (i.e. any complex social and environmental challenge). Analysis, prediction and control is a poor strategy for complex challenges, whereas there is growing indications that strategic approaches based on adaptation to real world context increases efficacy over time (Schreiber et al, 2022, Wolfe et al, 2018 - PDF, Lowe et al, 2022). This reality was particularly starkly highlighted during COVID-19, with a rapidly evolving situation which required Governments, Communities, Research and Businesses to engage in constant adaptation based on real world feedback.
[side note: interestingly, the dominant system recognises high failure rates of planning-based approaches (60-95% often cited), such as deficiet in communication, execution and participation, rather than pinpointing 'analysis & prediction' as the key challenges. Yet these same systems often turn to adaptive methodologies like Agile for projects which are subject to change over time.]
In this post, I want to expand a bit more on pathways to change, how experimentation aligns with the likes of living labs and mission-oriented innovation and then share a little about our thinking about designing experiments and capturing findings.
Systems can’t be controlled, but they can be designed and redesigned. We can’t surge forward with certainty into a world of no surprises, but we can expect surprises and learn from them and even profit from them. We can’t impose our will upon a system. We can listen to what the system tells us, and discover how its properties and our values can work together to bring forth something much better than could ever be produced by our will alone.
- Donella Meadows, 'Dancing with Systems'
Experimentation Process
I realise in the first post that it might have been useful to provide a quick overview of the experimentation process to ground these ideas in a view of the whole.
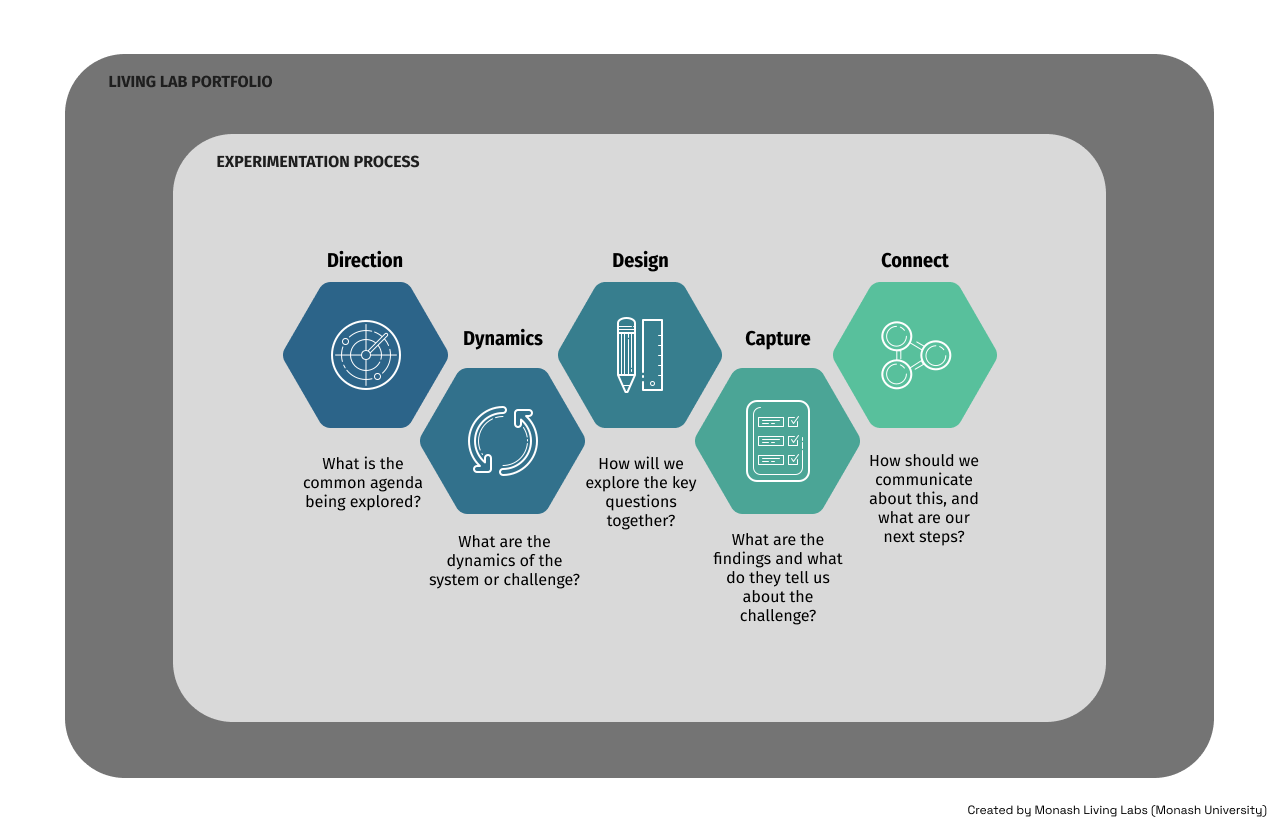
The core of this process is:
- Direction - maintaining alignment to a common agenda
- Dynamics - ensuring awareness of the system being explored
- Design - question-driven exploration and documentation of the approach
- Capture - reflection about the findings and their implications
- Connect - ensuring the connections of ideas, people and projects
It's worth saying this process isn't often a standalone aspect, instead it's aiming to bring rigour to the likes of Living Labs, Mission-oriented Innovation, and other collaborative processes aimed at influencing change. As such, it might look more like this for a Living Lab:
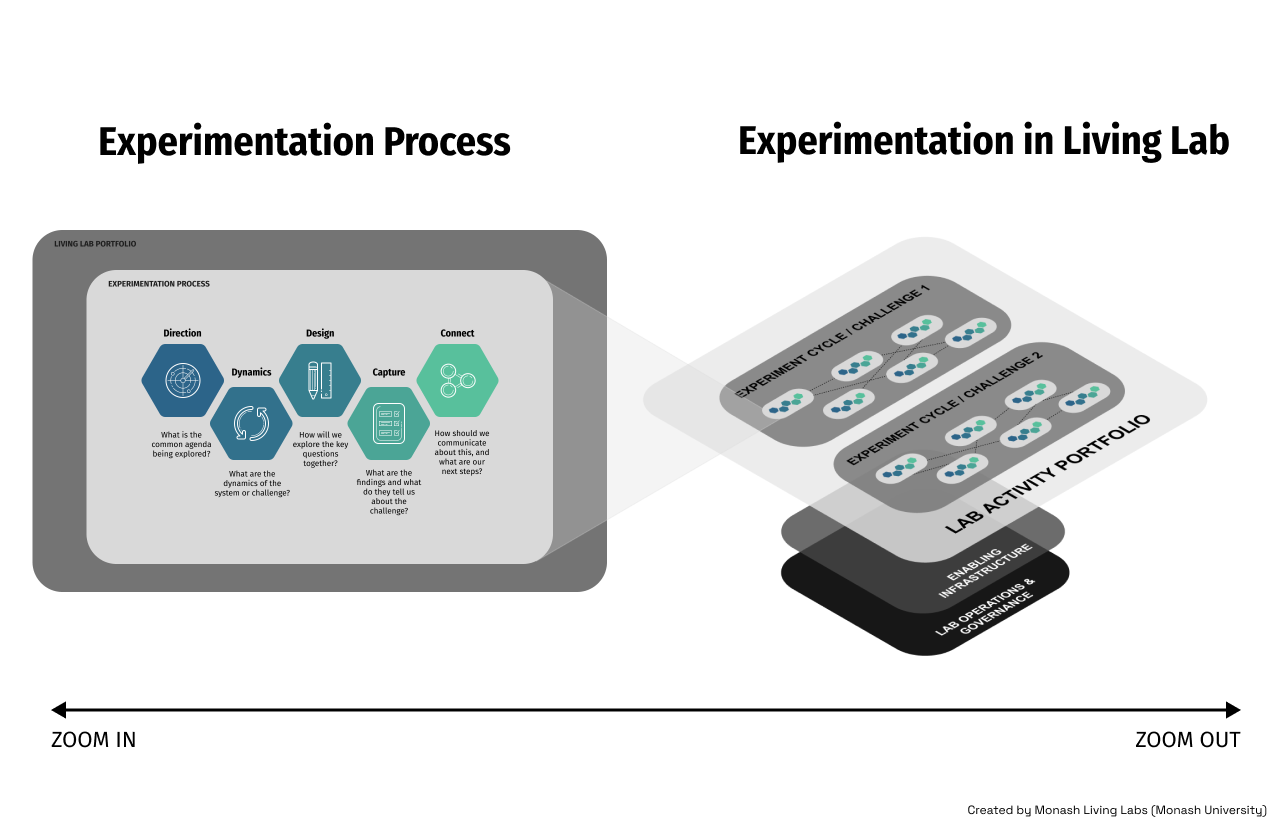
Likewise it might look more like this when embedded in Mission-driven Innovation:
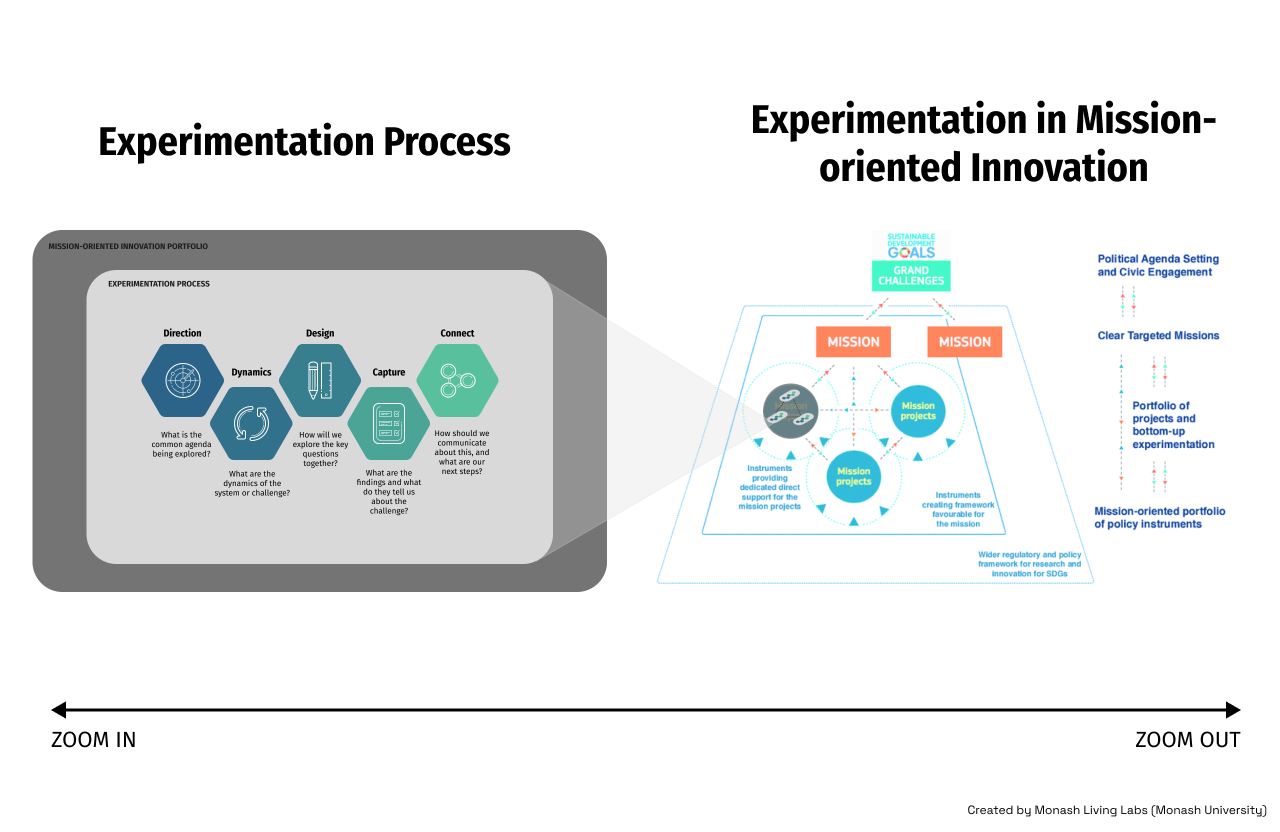
Pathways to change
In the previous post we spoke about (Step 1) Direction and started to talk a little about (Step 2) Dynamics too.
As a complement to understanding the dynamics of how change happen, I find it useful to factor in some discussions and understanding about pathways to influence those dynamics.
These are not dissimilar to how Meadows thinks about 'systems leverage' back in the previous post, and influenced by the thinking of Stewart Brand's "Pace Layering":
Consider the differently paced components to be layers. Each layer is functionally different from the others and operates somewhat independently, but each layer influences and responds to the layers closest to it in a way that makes the whole system resilient.
From the fastest layers to the slowest layers in the system, the relationship can be described as follows:
Fast learns, slow remembers. Fast proposes, slow disposes. Fast is discontinuous, slow is continuous. Fast and small instructs slow and big by accrued innovation and by occasional revolution. Slow and big controls small and fast by constraint and constancy. Fast gets all our attention, slow has all the power.
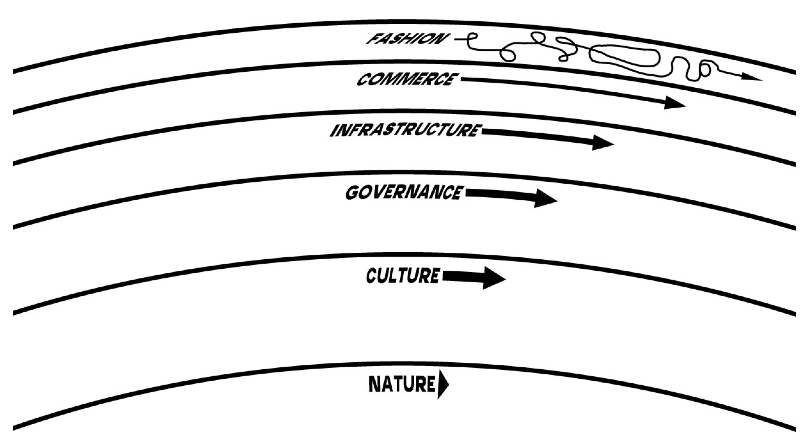
In Pace Layering, Brand and Eno presented a flexible model for understanding the inter-relation of change dynamics.
I find this useful to think about in broad terms, but when devising experimentation to influence these, I always find I want more detail, so here's a quick sketch of some of the specific elements I might target as 'pathways to change':
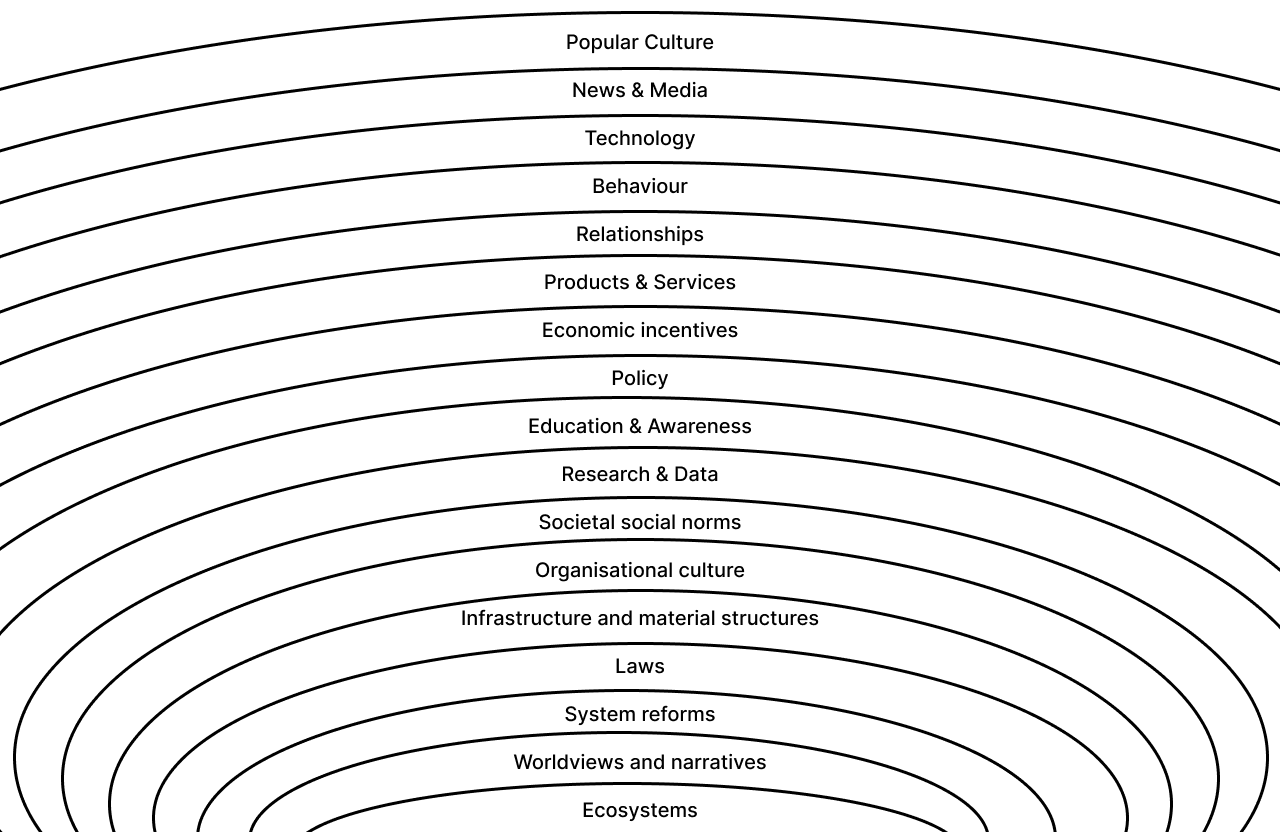
I know, it's a lot to take in - but when we're designing experiments and contemplating how we're seeking to influence change, we have many options to assess. The process of designing the experiment, often in a group, is valuable to help define some of these critical aspects to align around - so forcing ourselves to think through the boundaries brings greater precision and rigour.
Designing Experiments
The third step in the process is to move from the assumptions about how we think change will happen, into designing an experiment to test those assumptions.
This is where the rubber hits the road, and all too often the bubble of creativity and innovation can take over, and the rigour falls away. Instead, over 15+ years of working in this space, I've found a repeatable process, well documented, is critical to sustaining engagement, participation and learning over time.
The Experiment Design Canvas
This canvas represents several steps to support really thinking through what we're trying to achieve, the methods we'll use, and how we make sure we capture what we need to learn about. Time and energy are always limited, so ensuring we're spending resources well, increasing efficacy of experiments over time, whilst properly bringing people on the journey with us.
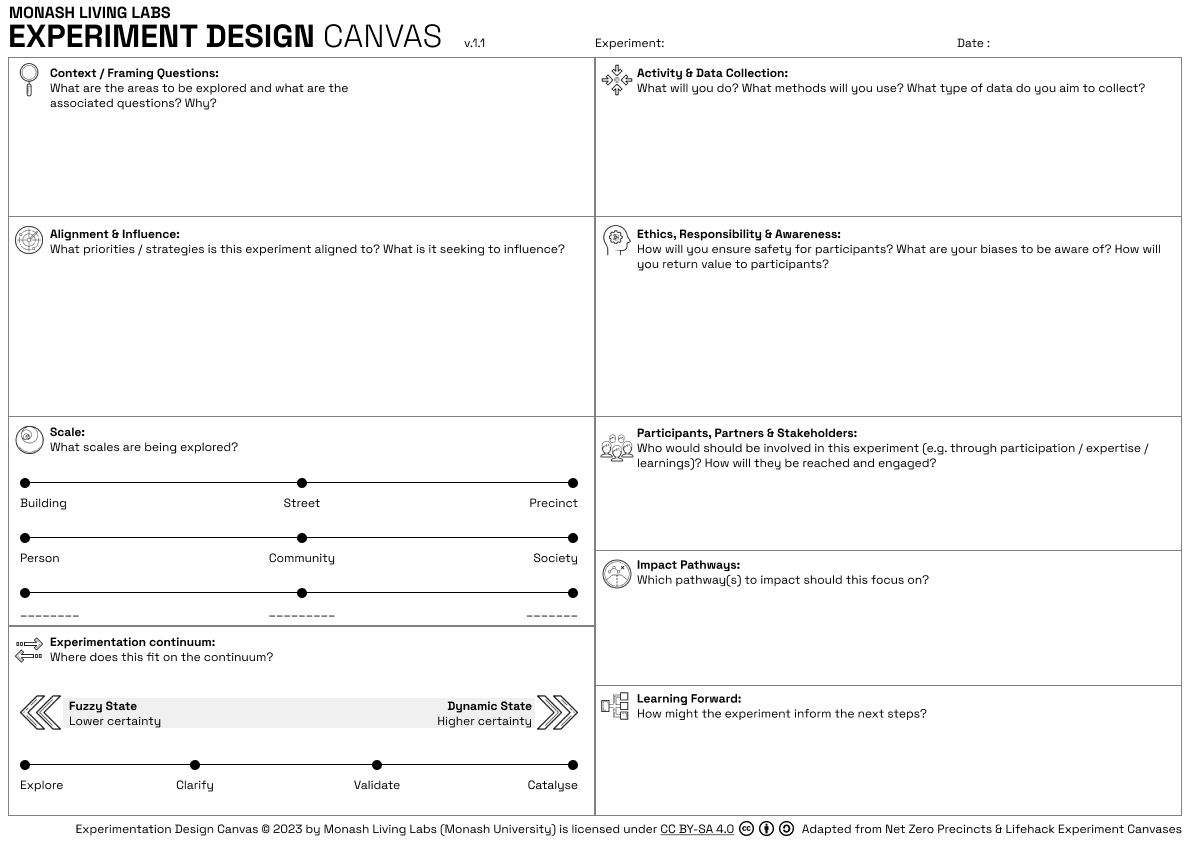
Obviously there's some nuance in how you approach unpacking these insights, it's not just a matter of scribbling down the first thing that comes to your mind. Hence, at Monash, we're building a workshop playbook and specialised capability alongside these canvases, to support effective experimentation over time.
Once the experiment is designed, of course - it has to be run. That could take anything from an hour or two, to several weeks or months in some cases. From our experience, starting smaller and building compelxity and intensity of experiments over time is the best approach to people new to this style of work.
This is obviously quite different to a Planning-centric approach, where you might outline 10 elements of a change initiative at the stage the project was funded, which often then affords little ability to adapt to findings and insights as the project rolls out. As mentioned in the intro, this is one of the principles of adaptive governance which implies a continual process of learning and adapting.
Capturing Experiments
The fourth step is to capture the results - be it qualitative or quantitative data, or in ways which aim to maintain the context, such as Storywork, Place-based methods or Warm Data.
Likewise reflecting on what the implications are, and what that means for the broader portfolio is also important.
The Experiment Capture Canvas
Just as we shared a canvas for designing, we also developed a canvas for capturing. Again, this is a place to document a process, and may need to involve both experiment participants and stakeholders to fully unpack.
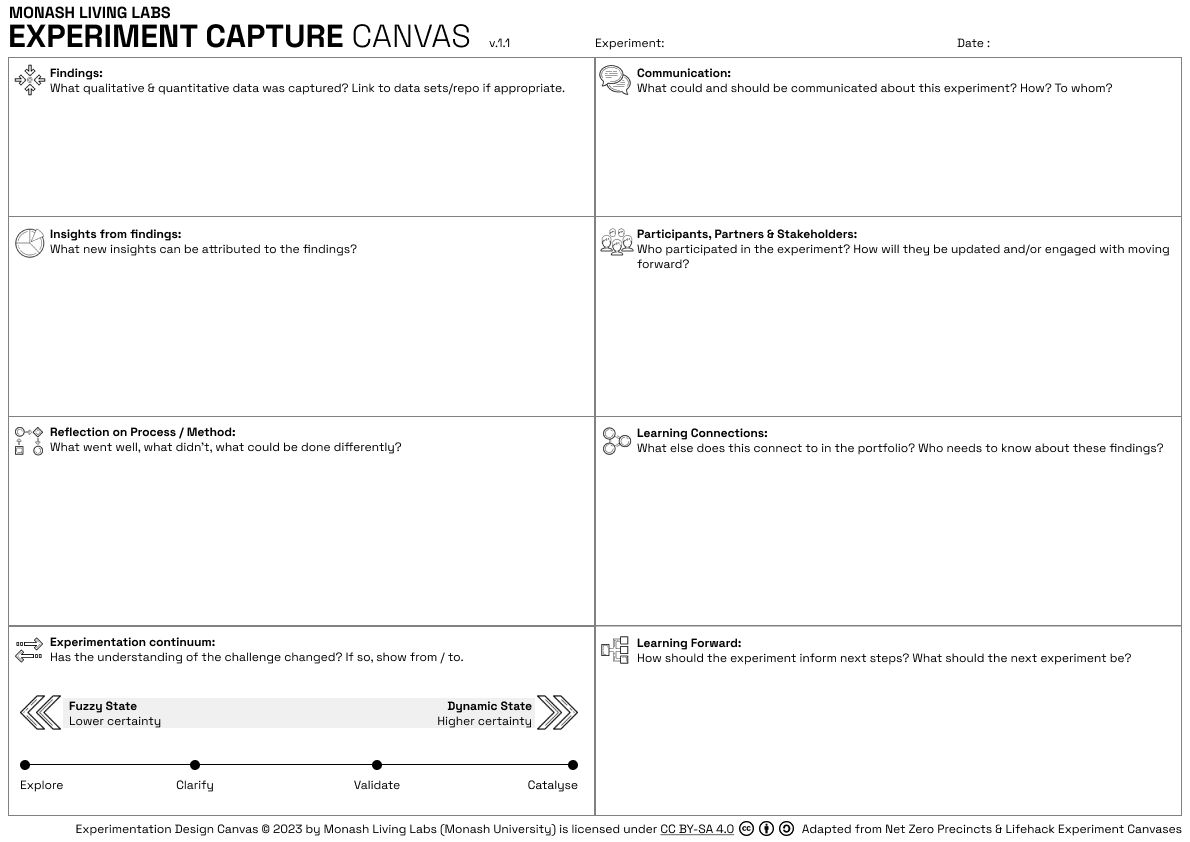
So, in this post we've explored an Experimentation Process, and how we might understand and design for certain pathways to change. We delved into the detail of Experimentation Design and Capturing Findings.
In the next posts in the series, we will talk about the final step in the process (Connect), what to do with all the experiments (developing a system), reflect on mindsets and capabilities, perhaps even explore leading edges like 'AI for knowledge management & activation', and take a dive into a practical example of experimentation in action in the Net Zero Precinct space.
As always, I very much welcome feedback, comments, reflections, challenges - I use this space to explore and think out loud. I'm not presenting a methodology™ because I'm learning how to do this, like everyone else, so please do get in touch if you're figuring out this journey too.
Comments ()